What Is Liquidity And How Does It Affect Prediction Markets?
By Ben Golden on December 16, 2015
Our sites use a popular prediction
market algorithm called LMSR to determine how markets adjust when someone makes
a forecast, and how user scores are affected by making correct and incorrect
forecasts. When creating a LMSR market, administrators must set something
called the liquidity parameter, or b parameter. There's been a fair bit of
discussion about managing liquidity in prediction markets, and in this post
I'll explain why liquidity matters, how to manage it, and the benefits of
different approaches.
Liquidity refers to how much something can be bought or sold in a market without affecting its price. As an example, I'm going to show possible forecasts in three different questions on Alphacast. In each case, my plan is to buy roughly the same amount in each market, and show how each forecast affects market forecasts.
Question 1:
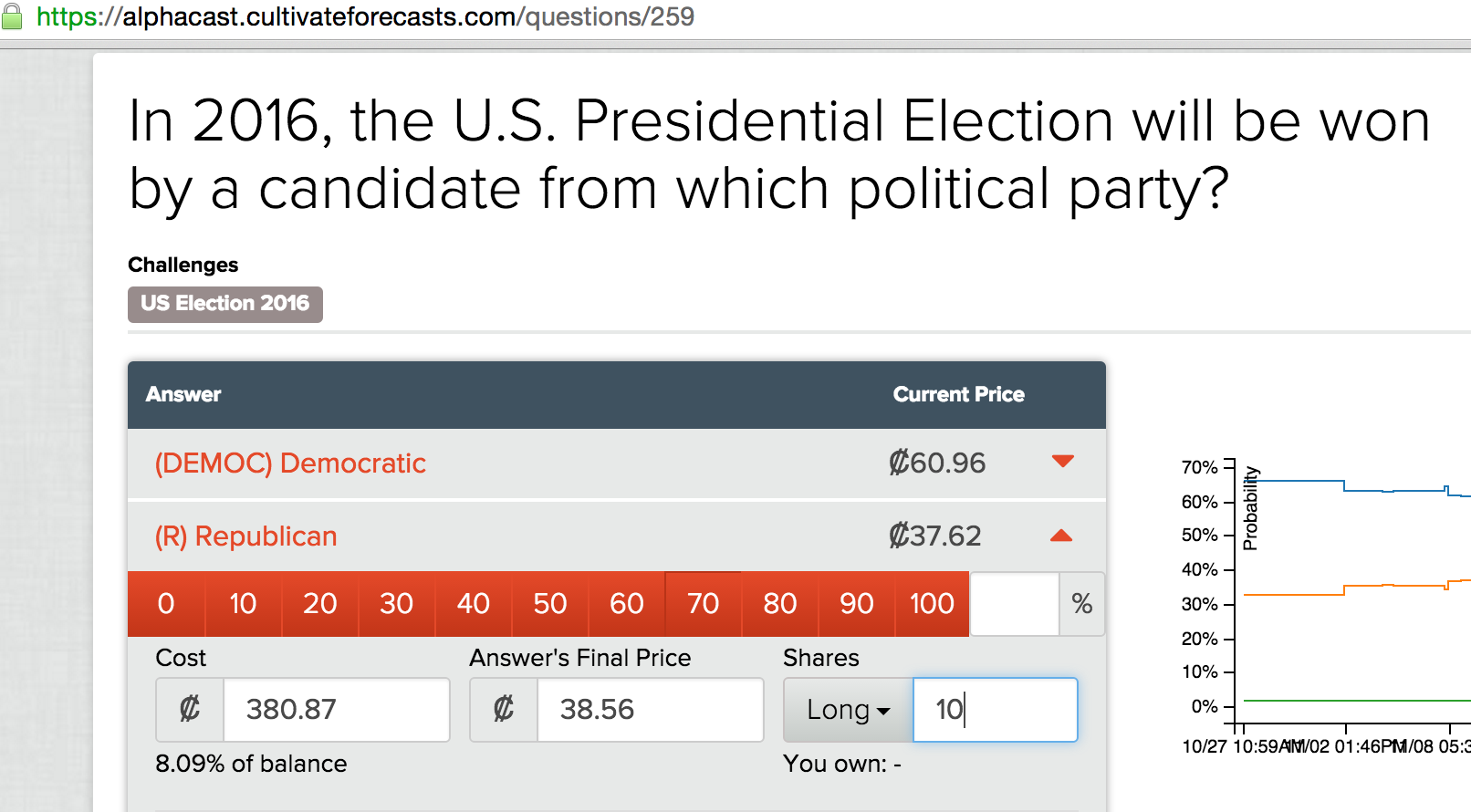
Question 2:
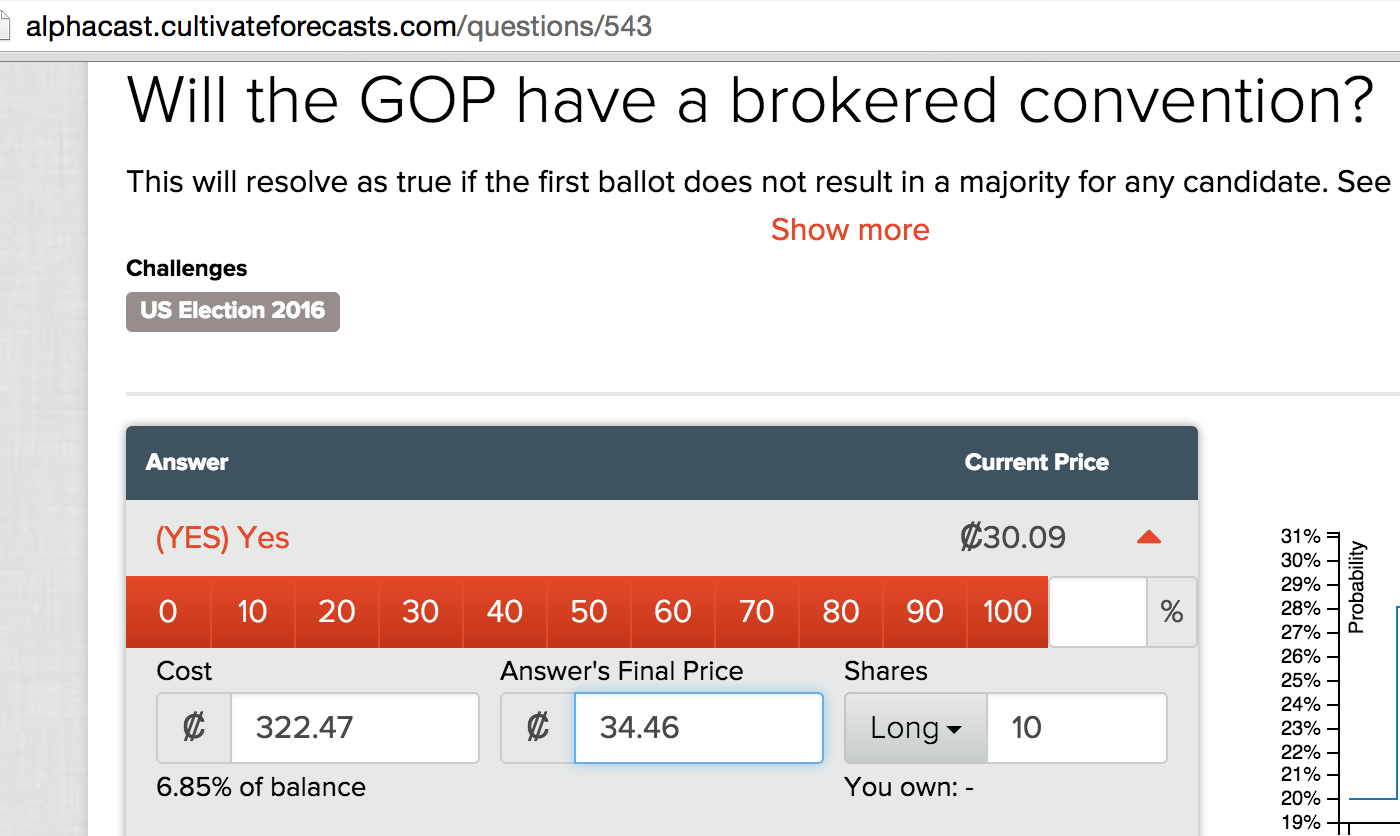
Question 3:
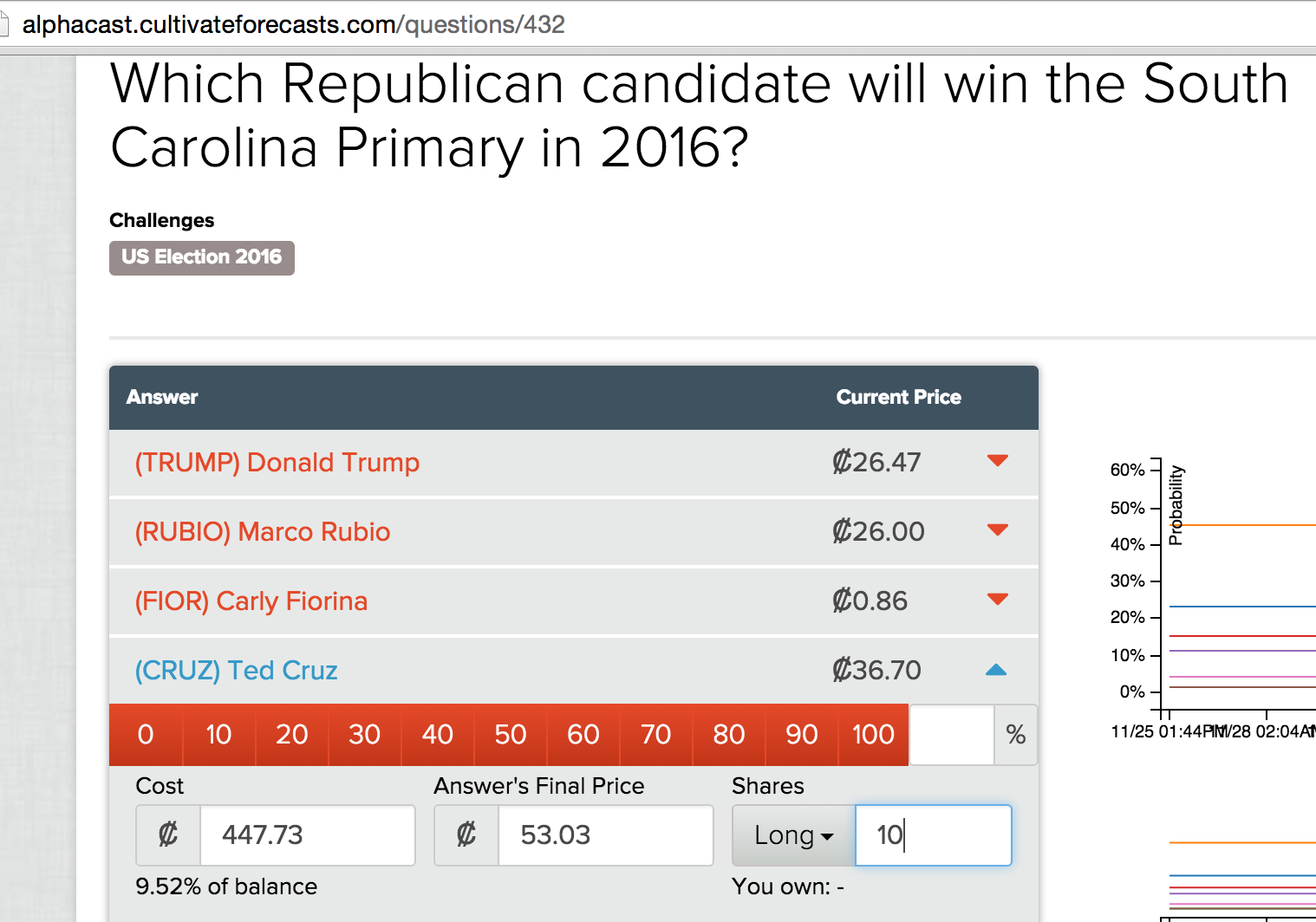
I'm using our trader mode to buy 10 shares in each forecast. The starting probabilities for each answer I'm forecasting are similar (between 30 and 38), but note that the final price in each case is changing by a vastly different amount. In question 1, the probability changes from by about 1%, from 37.6% to 38.6%. In question 2, it changes by about 4%, and for question 3, it changes by 26%!.
These differences are caused by the fact that each question has a different liquidity parameter. Liquidity is really important to forecasters looking to increase their score. Having too little liquidity makes markets seem meaningless because any user can change any price with very little risk, while having too much liquidity can flood the market with easy money, creating an entirely different set of problems. The liquidity parameter determines how much probabilities move after a forecast, with higher liquidity parameters leading to smaller changes and lower liquidity parameters leading to larger changes. After some experimentation we determined that Question 1's liquidity (250) is probably too high, Question 3's (15) is too low, and Question 2's (50) is, for now, our Goldilocks liquidity. We're not changing existing questions, but going forward we'll mostly be using 50 as our liquidity parameter.
In traditional double auction markets, such as the stock market or PredictIt, you also see varying liquidity. Here are two questions on PredictIt:
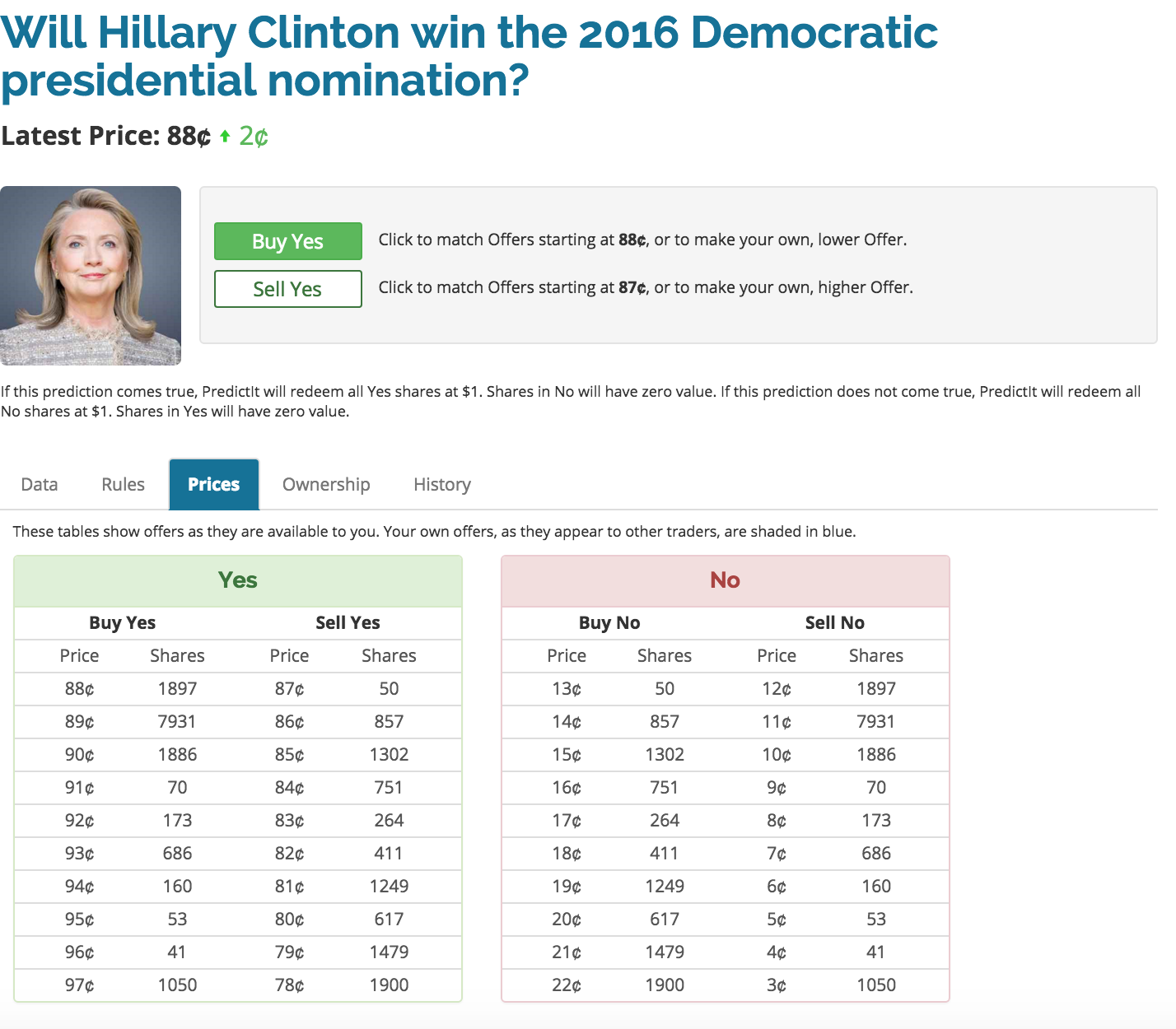
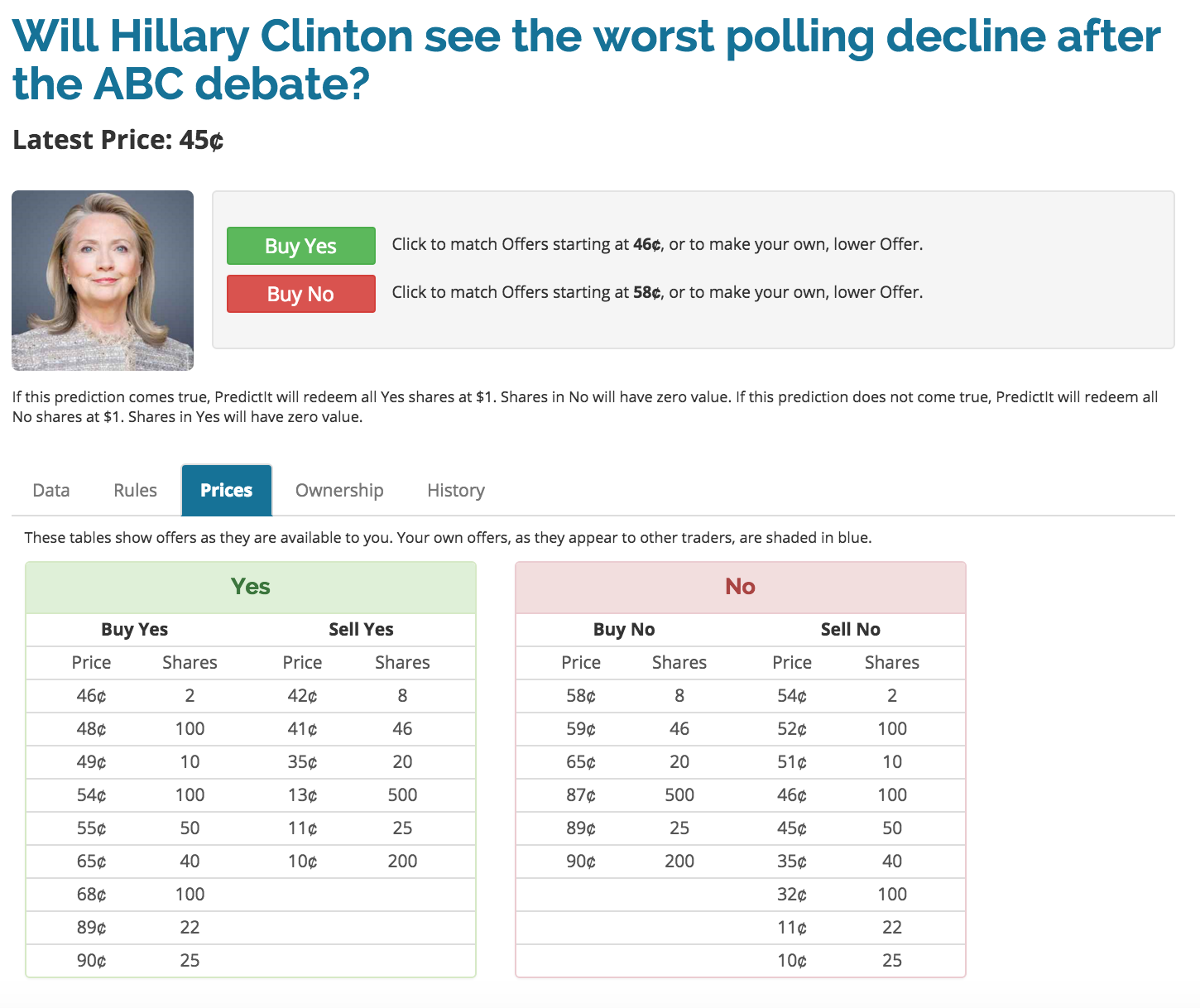
The first market (for the nomination) has much greater liquidity than the second. You see this because there are more shares available at prices closely clustered together--in the first market, buying 10,000 shares would move the forecast from 88¢ to 90¢, whereas in the second, buying about 400 shares moves the forecast from 45¢ to 68¢.
Whereas LMSR markets set liquidity to a certain value, double auction markets let users determine liquidity. More popular questions like who will win a presidential nomination tend to have greater liquidity, whereas asking who a debate will affect polling may have lower liquidity. One of the key advantages of using LMSR is guaranteeing enough liquidity to make markets meaningful--double auction markets can literally have zero liquidity (if no users have made orders).
But a key question remains as to what the liquidity should be for a question, whether it should be constant across questions, and even within questions. There's been some interesting research into prediction markets with varying liquidity, which is being explored by the crypto-currency prediction market Augur. In a future approach, I'll discuss this proposal, and some other ideas about how to manage liquidity in prediction markets.
Did you know that you can sign up for free at AlphaCast? If you liked this post, you can follow us on Twitter at @cultivatelabs, and sign up for our monthly newsletter.
Ben Golden/@BenGoldn is an Engineer at Cultivate Labs.